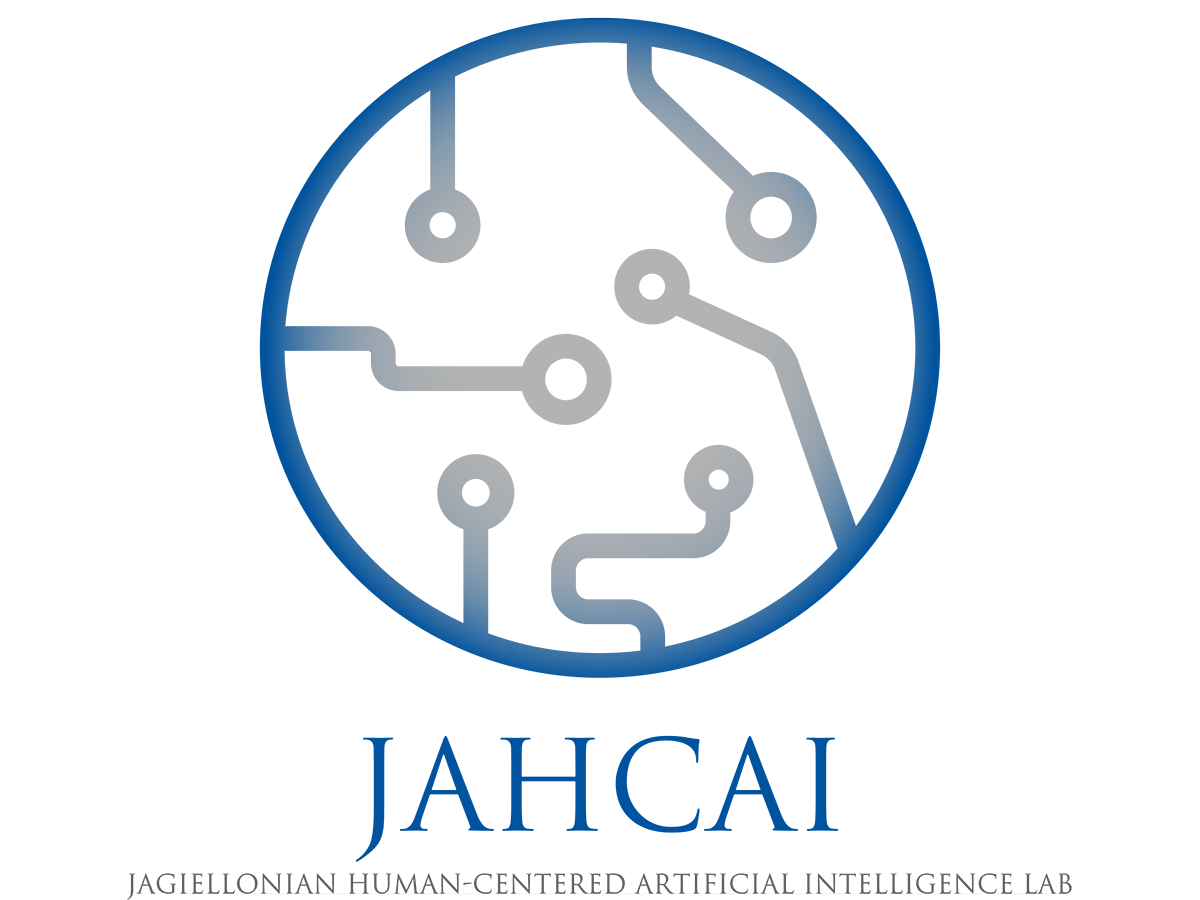
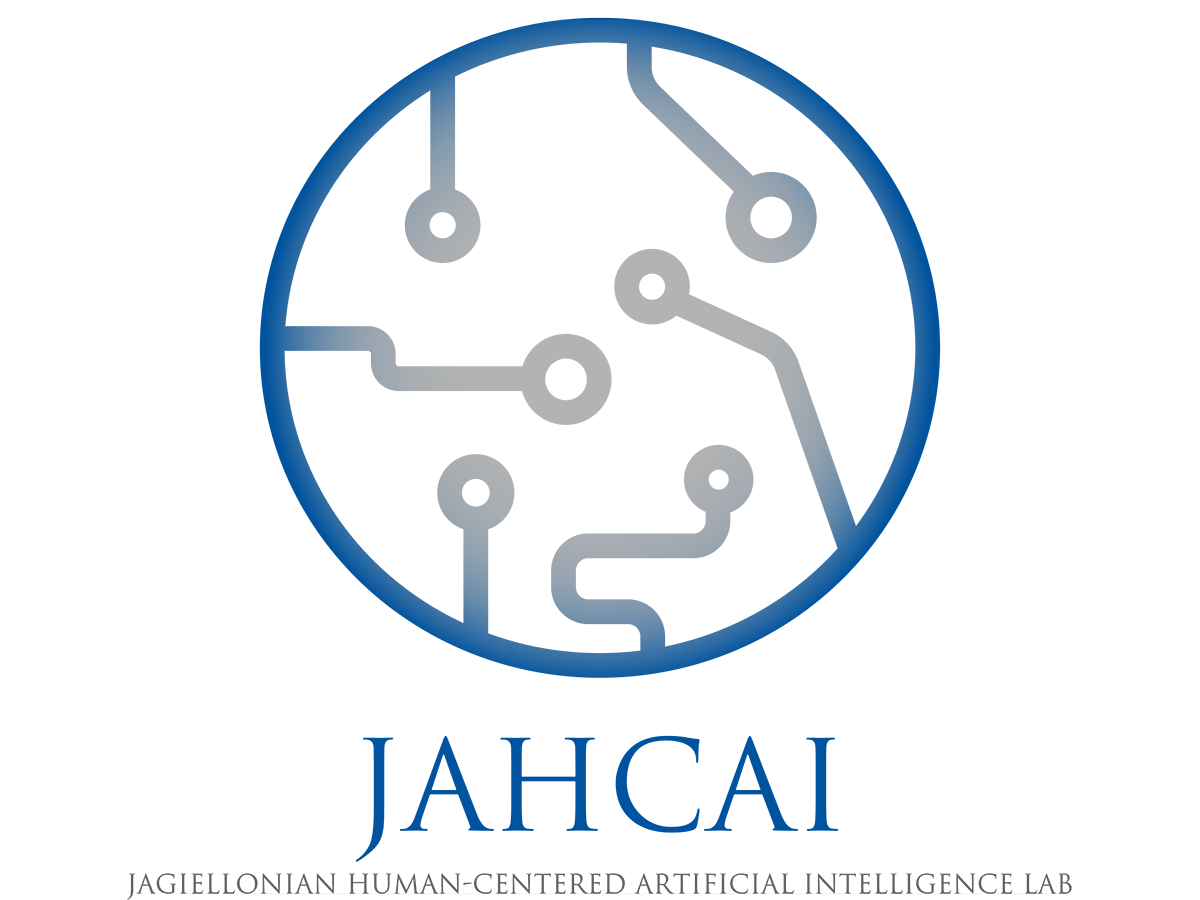
New publication authored by the members of JAHCAI - Szymon Bobek and Grzegorz J. Nalepa, along with Jakub Jakubowski and Przemysław Stanisz.
Members of the Centre have recently published an interesting work on enhancing explainability of the AI in predictive maintenance. In the article, published by the prestigous journal Sensors, the authors took up the issue of lack of good quality labelled data used by the automated systems used in predictive maintenance. They present the aplication of unsupervised learning using a variational autoencoder, with application of explainability methods for the purpose of understanding the model's predictions. They conclude that " the variational autoencoder slightly outperforms the base autoencoder architecture in anomaly detection tasks" and that "the information obtained from the explainability model can increase the reliability of the proposed artificial intelligence-based solution".
If you are interested in finding out more specifics of the research, feel free to read the article, published in Open Access, under this link.